It would appear that to accept statistics at face value is a fool’s bargain. People are obviously swayed by the media hype, which assures them that the casualty numbers of the virus are statistically significant and that adverse reactions to the vaccines are statistically negligible. But are they?
Sucharit Bhakdi, formerly of the Max Planck Institute of Immunobiology and Epigenetics, currently chair of Medical Microbiology at the University of Mainz and co-author of Corona False Alarm? shows how Germany’s federal government and research agency for disease control, the RKI—the country’s counterpart of the CDC in the U.S.—had juggled the numbers. The RKI, he writes, “calculated that 170,000 infections with 7000 coronavirus deaths equals a 4% case fatality rate.” The problem is that “the number of infections was at least ten times higher because mild and asymptomatic cases had not been sought and detected. This would bring us to a much more realistic fatality rate of 0.4%.”
Additionally, deaths from other causes were folded into the mortality count. A true statistical correction “would yield an estimate of between 0.24% and 0.26%.” Sucharit wryly provides a hypothetical example. “If I drive to the hospital to be tested and later have a fatal car accident…I become a coronavirus death. If I am diagnosed positive for coronavirus and jump off the balcony in shock, I also become a coronavirus death.” Statistically speaking, it’s a good gig if you can get it.
The trim-and-shuffle seems to be par for the course. For example, the FDA’s report on Pfizer’s vaccine counts “3410 total cases of suspected, but unconfirmed covid-19 in the overall study population.” Nonetheless, the pharmaceutical company reported “a 95% relative risk-reduction figure.” As Dr. Peter Doshi explains in the British Medical Journal : “With 20 times more suspected than confirmed cases, this category of disease cannot be ignored simply because there was no positive PCR test result.” Bundling in both the suspected and confirmed cases, Doshi notes, would drop “the 95% relative risk-reduction figure down to only 19%.”
A relative risk reduction of 19 percent is far below the 50 percent effectiveness threshold for authorization set by regulators. And even the 19 percent tally assumes that the data are veridical. Bluntly speaking, what these interested participants are doing is eliminating unfavorable factors.
The Defender (Children’s Health Defense) points out that when one does the real math, the Pfizer clinical trial numbers showed that “the risk reduction in absolute terms [was] only 0.7%, from an already very low risk of 0.74% [in the placebo group] to a minimal risk of 0.04% [in the vaccine group].” Dividing 0.7 by 0.74 is the mathematical calculation that produced the touted “95% effective” number. The result has been corroborated by the quality virological journal Vaccines, which reports that the absolute risk reduction is less than 1 percent. Clearly, Pfizer cooked the books. The math was right, so far as it went—which was not very far—but the statistical implications were misleading. What cannot be denied is that, by any metric, vaccine efficacy remains low. It seems evident that data collection is often intended to paint the wished-for statistical canvas.
Equivocal statistical lattices are the optimal way of actually suppressing inauspicious or compromising information. A recent case in point: the CDC took steps to censure unfavorable data concerning breakthrough infections from their reporting systems. In fact, as The Guardian reports, the CDC is now adjusting its testing protocols to reduce the number of “breakthrough cases” by lowering test cycles for vaccinated people and raising them for the unvaccinated. Higher test cycles will pick up junk virus and dead virus, creating a disease “that can appear or disappear depending on how you measure it… This is a policy designed to continuously inflate one number, and systematically minimize the other. What is that if not an obvious and deliberate act of deception?” The ginned-up statistical count will be through the roof. The Guardian concludes: “If these new policies had been the global approach to ‘Covid,’” that is, employing reasonable test cycles for all participants, “there would never have been a pandemic at all.”
In effect, the tendency is to boost the casualty count with respect to the virus and to reduce it with respect to the vaccines. That is how the game is played by disreputable proponents of progressivist, “social justice,” and authoritarian causes, of which the most prominent today is COVID prevention. A medicated statistic is nothing less than a damn lie. The distinction is moot, the only difference being that between an outright falsehood and a clever dissimulation. Damn lies and statistics are among the best weapons our so-called “experts” can deploy. And it must be admitted that these are effective instruments of subterfuge and control, owing both to their deceptiveness, their air of authority, and their volume.
In The Data Detective, Tim Harford puts a more benign slant on the issue, pointing to inevitable selection bias in statistical claims. There are huge disparities in such claims since, for various reasons, many facts may not be recorded. Sometimes, what he wittily calls “premature evaluation” plays a role, i.e., early overcounting, undercounting, or counting the wrong items. Harford shows how easy it is for researchers to get things wrong. Researchers may not be duplicitous—or at least, not always or often—but merely sloppy.
Renowned Stanford University epidemiologist John Ioannidis is less sanguine. Ioannidis predicted in a landmark 2020 research paper that we would see exaggerated estimates regarding COVID cases, infection spread, and mortality rates—in other words, false research findings. As he writes, “the majority of modern biomedical research is operating in areas with very low pre- and post-study probability for true findings.” Moreover, “conflicts of interest tend to bury significant findings.” Ironically, data relationships “reaching formal statistical significance…may often be simply accurate measures of prevailing bias” rather than of objective facts. In another paper written at approximately the same time, Ioannidis speculates that the pandemic may be “a once-in-a-century evidence fiasco.”
Instead of heeding Ioannidis’ warnings, the official echelons and media platforms chose to follow the doomsday statements, contradictory evidence, and “voodoo mitigation efforts” promulgated by the increasingly distrusted Dr. Anthony Fauci, as Steve Deace and Todd Erzen amply detail in their cleverly titled, must-read volume Faucian Bargain. They show how data can be routinely finessed to support prior assumptions and to erect statistical scaffolds for problematic arguments and false conclusions. Samples are regularly used either to swell or shrink averages. If one instrument doesn’t work, switch to another.
This is standard-issue practice, a form of statistical gerrymandering, manipulating the boundaries of a given data-set in order to produce a desired result. Pandemicians have not only, to use a popular phrase, continued to “move the goalposts,” they have performed the magic trick of moving the whole football field. For example, Fauci has constantly changed his “herd immunity” percentages from 60 to 70 to 75 to 80 to 85 and even 90 percent. Similarly, the WHO suddenly and for no sound reason changed its definition of herd immunity several times. The instances I have flagged above are merely illustrations of systematic deceptive protocols to shore up spurious or hypothetical clinical claims.
It has been said that if the facts don’t fit the theory, change the facts. Analogously, if the statistics don’t accommodate the wished-for results, massage the statistics. The game is rigged from the start.
—————————
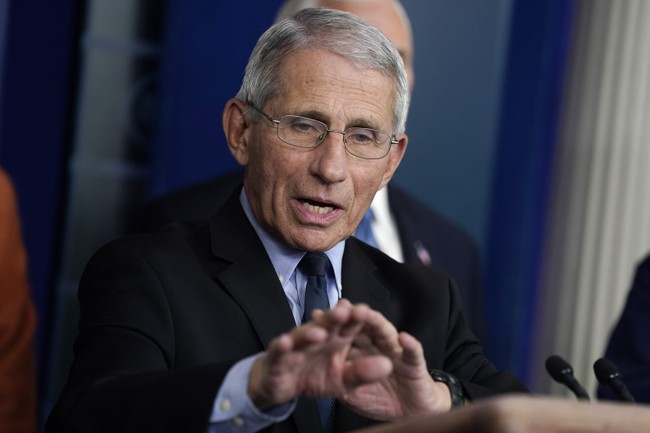
Aside from those mentioned within, I cite three important books that deal, wholly or in part, with the nature of data manipulation and institutional guile: Liberty or Lockdown by Jeffrey Tucker, The Price of Panic by Douglas Axe, William Briggs and Jay Richards, and The FEAR-19 Pandemic: How lies, damn lies and statistics created a pandemic of fear by Tommy Madison. They make for essential reading.
Join the conversation as a VIP Member